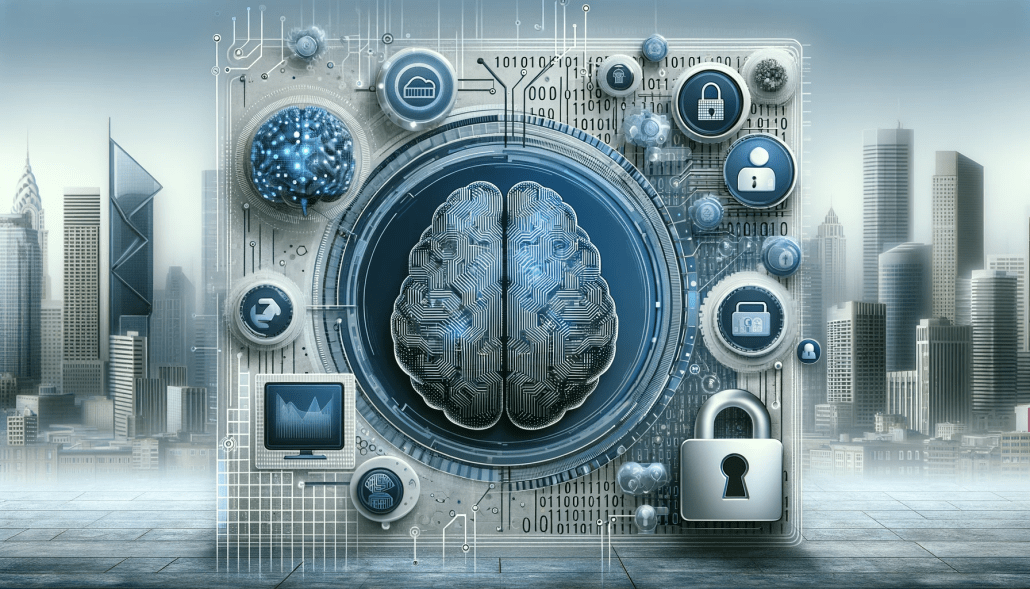
In an era where Artificial Intelligence (AI) reshapes financial services, dramatically improving operations and revolutionizing customer experiences, the imperative of data privacy and security has emerged as a number one priority for business leaders. At Data Management Group (DMG), we’ve been at the forefront of this shift, collaborating with leading financial entities to navigate and neutralize AI-related data risks. This guide distills our insights, offering a blueprint to safeguard your company’s data and your customers’ data against emerging threats.
In this post, we’ll delve into the potential vulnerabilities introduced by AI and provide recommendations institutions can put in place to ensure robust data protection. Subsequent posts in this series will cover concerns around implementing AI in other areas, like bias and fairness, regulatory compliance, data quality and accuracy, and transparency and accountability.
The AI Paradox: Potential vs. Pitfalls
AI holds immense promise, from predicting market trends to offering personalized banking solutions. However, its very nature, which involves sifting through vast datasets, can inadvertently introduce vulnerabilities. Unauthorized access, potential breaches, and misuse of data are genuine concerns. Financial institutions must proactively counter these threats.
Here are 5 keys to help your company get all the benefits of AI while at the same time neutralizing threats around data security and privacy.
1. Develop and Adhere to AI Principles
The foundation of any AI-driven initiative in the financial sector should be built on sound AI principles. These principles serve as a compass, ensuring that AI models are transparent, fair, accountable, and designed with privacy in mind. By embedding these principles into the AI lifecycle, from data collection to model deployment, financial institutions can mitigate risk and uphold their commitment to data protection.
DMG’s Recommendations: We recommend that companies outline a set of AI principles that can be used to guide how LLMs are trained on data and provide guardrails around their outputs. This includes:
- Establishing a set of AI principles that are tailored to your organization’s values and goals.
- Ensuring that these principles are communicated across teams and are at the forefront of any AI project. Principles often include a commitment to data privacy, ensuring AI is trained in a way that prevents bias, and adding requisite safeguards to ensure data is only accessible by those people or systems who truly need it.
2. Establish Comprehensive AI Governance
Beyond principles lies governance — a robust framework that encompasses policies, procedures, and oversight. Effective governance ensures that AI models align with organizational goals and regulatory standards, offering clarity on data usage, model interpretability, and unforeseen outcomes.
DMG’s Recommendations: To ensure that your organization has appropriate checks and balances in place, we recommend that organizations:
- Implement a multi-disciplinary AI governance committee, comprised of data scientists, legal experts, and business leaders.
- Conduct a regular review of existing and proposed AI initiatives, ensuring they align with established principles and address emerging concerns.
- Provide avenues of recourse for the committee to raise red flags if and when they encounter potential activity that runs counter to the organization’s principles.
3. Bolster Security by Fortifying the Data Itself
AI models, by their nature, process vast amounts of data, making them potential targets for cyber-attacks. Ensuring the security of these models, the data pipelines feeding them, and the infrastructure supporting them is paramount. While AI can accelerate data processing, enhance insights, and automate tedious tasks, it can also introduce vulnerabilities if not secured properly.
These vulnerabilities include threats like data poisoning, where malicious actors can introduce “poisoned” data into the training set, causing the model to learn incorrect behaviors. Model inversion and extraction, where attackers can use the outputs of an AI system to reconstruct valuable information about its training data or even replicate the model itself, is another threat.
DMG’s Recommendations: We work with companies to regularly conduct AI-specific vulnerability assessments, implement end-to-end encryption, establish robust access controls, and set up real-time monitoring to detect and thwart potential threats. Among the specific steps we recommend companies take are:
- Robust model training to recognize and resist adversarial inputs, making them more resilient to such attacks.
- Data validation, which ensures that the training data is clean, relevant, and free from anomalies. Companies should regularly update the training set to account for new data patterns.
- Differential privacy techniques should be implemented to ensure an individual’s data cannot be reverse-engineered from the AI’s output, thereby adding a layer of privacy protection.
- Regular security audits should be performed to evaluate the AI system for vulnerabilities. Employ red teams to simulate real-world attacks and gauge the system’s resilience.
- Continuously monitor and log AI operations. Any anomalies or unexpected behaviors should trigger alerts for immediate investigation.
4. Implement PII Data Security and Controls
Personally Identifiable Information (PII) is a goldmine for malicious actors. Financial institutions must implement stringent controls to ensure that PII is not only stored securely but is also processed in a manner that respects individual privacy. Large companies handle vast amounts of PII, which is often stored in disparate systems, making it difficult to track and secure.
DMG’s Recommendations: There are a number of approaches we recommend to ensure security of PII. Companies should always:
- Anonymize or pseudonymize PII data before feeding it into AI models
- Implement data masking techniques and ensure that access to PII is strictly on a need-to-know basis. Data masking conceals the original data with modified content (characters or other data) that is structurally similar to the original data. This is especially useful for testing and training environments.
- Encrypt PII, both in transit and at rest. This is a vital step to ensure that even if data is accessed, it remains unreadable.
5. Set Up PII Network Screening
Ensuring the security of the network through which PII data flows is crucial. Any weak link in the chain can lead to potential breaches, undermining trust and leading to regulatory repercussions. Modern enterprises often have intricate networks that span multiple regions, cloud providers, and on-premises data centers. As networks become more complex and interconnected, the challenge of ensuring PII is securely handled within these networks grows exponentially. The sheer volume of network traffic can also make real-time screening a resource-intensive task.
DMG’s Recommendation: As with PII data security and controls, there are a number of approaches financial institutions can take to ensure the safety and security of networks that handle PII. We recommend:
- Deploying advanced network screening tools that monitor data flow in real-time
- Implementing intrusion detection systems and ensuring regular patching of network vulnerabilities
- Setting up Deep Packet Inspection (DPI), which involves examining the content of network packets to identify any PII data being transmitted. DPI can detect PII even if it’s not part of regular database traffic, such as in emails or file transfers.
- Network segmentation, or creating isolated segments within the network to ensure that PII is only accessible and transmissible within designated secure zones.
Data Privacy and Security Around AI is Difficult But Far From Impossible
While AI introduces a new dimension of concerns in the realm of data privacy and security, with a principled approach, robust governance, and stringent security measures, financial institutions can harness the power of AI without compromising on their commitment to data protection. As the financial landscape continues to evolve, staying proactive and informed will be the key to navigating the challenges and opportunities that lie ahead.
If your firm is working through some of these challenges and would like outside expertise ensuring you can take advantage of all that AI has to offer while mitigating the risks, please reach out to us today for a complimentary consultation.