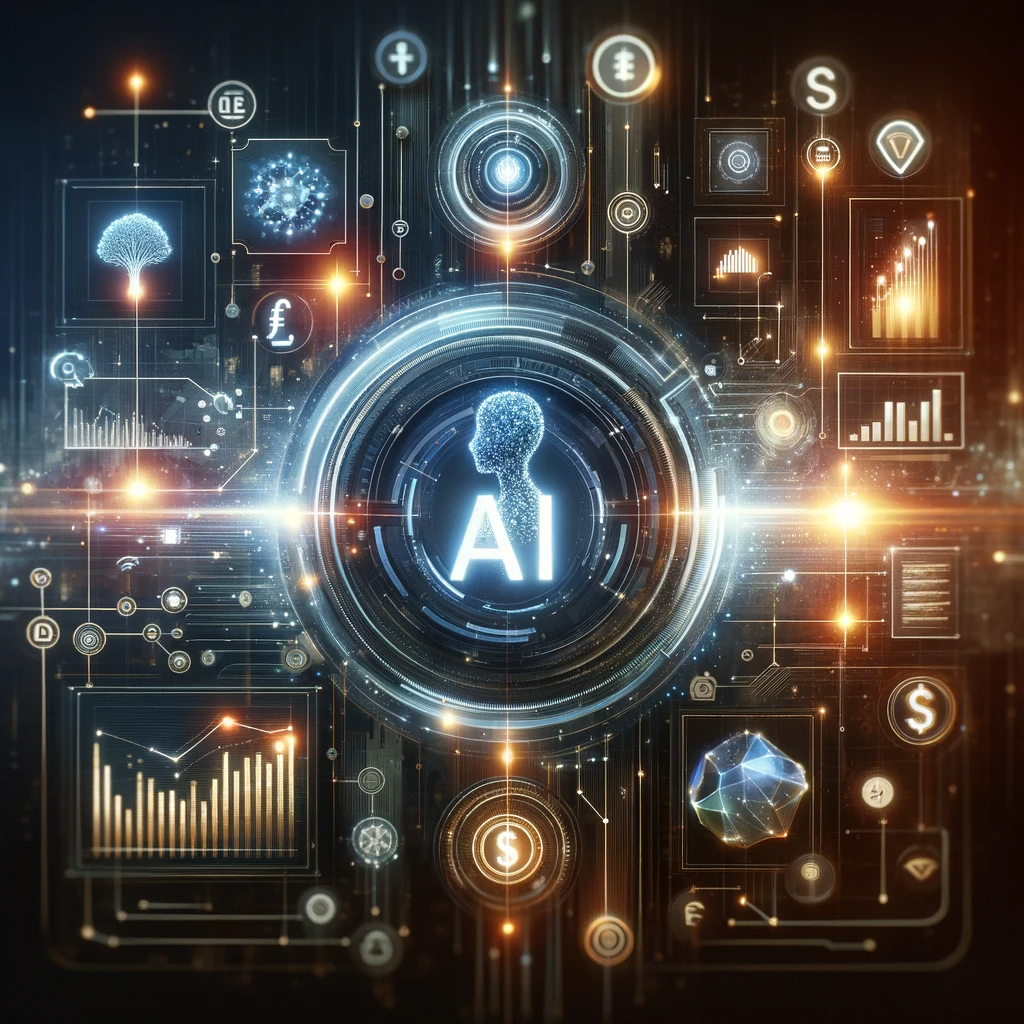
The rapid adoption of artificial intelligence (AI) in Financial Services has underscored the immense value AI can bring to data-driven decision-making. However, the reliability of AI is only as good as the quality of the data it is trained on. Poor data quality or inaccuracies can lead to misguided decisions with far-reaching consequences. To address this, it is crucial for financial enterprises to embrace AI Data Quality and AI Data Governance tools, enhancing the integrity of Critical Data Elements and ensuring cost-effective scalability in their AI initiatives.
At Data Management Group (DMG), we recognize that data is the bedrock of AI reliability. Our suite of solutions is designed to empower your organization to maintain high-quality data, allowing AI to deliver more accurate and dependable business outcomes.
In this post, we offer our recommendations on how companies can improve data quality and accuracy, ensuring the maximum benefit from their AI initiatives. Our recommendations include:
- Ensuring Scope and Definition of Critical Data – What you consider Critical Data Elements will impact data integrity
- Leveraging Advanced AI Data Quality Tools – Take advantage of AI tools to optimize data quality
- Employing AI-Powered Data Governance – Bring AI into your data governance processes to ensure your processes evolve as needed
Ensure the Scope and Definition of Critical Data
In large enterprises, data quality can be notoriously inconsistent. Companies traditionally identify critical data types and manage those data pipelines more rigorously, or they employ Master Data Management (MDM) tools to ensure data reliability. However, this focus on “critical” data leaves a significant portion of data that may not be reliable, and AI systems often lack the capability to discern between reliable and unreliable data.
Additionally, there is significant cost associated with maintaining Data Quality, and so most organizations are forced to make a difficult decision to reduce the scope of Critical Data to only the Most Critical Data. Our recommendation is to leverage AI Data Quality methods and tools to be able to ensure the quality of the actual Business Critical Data (usually more data) at a lower overall cost.
DMG’s Recommendations: Use AI tools to cost-effectively increase your company’s Critical Data
We recommend that Financial Services companies:
- Adopt AI-enhanced data cleansing tools that can aid in detecting, correcting, and preventing errors in their datasets
- Review their definition of data quality and critical data and refine their data governance processes accordingly
- Institute a culture of data quality by providing regular training sessions for employees, reinforcing the importance of data accuracy in AI implementation
Leverage Advanced AI to Improve Data Quality
AI itself can be a solution to the problem of poor data quality. AI models can be utilized to identify and resolve data quality issues before feeding the data into business-level AI models. These tools are typically built in to the Data Governance tools and processes. The process of data cleaning includes removing duplicate data, detecting outliers in your data, forecasting missing data, and validating and verifying data.
DMG’s Recommendations: Evaluate and experiment with AI tools that can remedy poor data quality.
We recommend that Financial Services companies evaluate:
- Employing AI models designed to detect and correct inconsistencies and errors in data as part of the their Data Governance methods, processes, and tools
- Regularly subjecting your data to AI scrutiny, ensuring your data is clean, updated, and accurate before it serves any business-level AI models
- Incorporating automated data cleaning and preprocessing routines using AI, further raising the data quality standard
- Leveraging AI-driven data profiling to understand the quality of data at a granular level and improve it prior to using in business models
Employ AI-Powered Data Governance to Drive Strategic Decision-Making
Data governance traditionally involves setting policies and standards for data usage, ensuring compliance with regulations, and maintaining data quality. AI augments this process, introducing capabilities such as predictive analytics and real-time monitoring, turning static rules into dynamic, responsive frameworks that adapt as new data trends and patterns emerge.
DMG’s Recommendations: Incorporate AI into your data governance practices to ensure dynamic data quality
We recommend that Financial Services companies:
- Implement AI systems that can analyze vast datasets to predict potential data quality issues, flagging them before they impact decision-making
- Use machine learning models to continuously learn from data interactions, optimizing governance policies and ensuring they remain relevant in the face of changing regulations and business needs
- Automate compliance checks with AI algorithms that can sift through complex regulatory requirements, reducing human error and ensuring faster adaptation to new compliance challenges
Ensuring Reliable Data in Large Financial Institutions
Financial institutions are entrusted with making data-informed decisions daily. By proactively perfecting data quality and governance with advanced AI tools, these enterprises can both bolster their strategic decision-making capabilities and expand the universe of data that is considered CDEs.
At DMG, we are dedicated to guiding financial services through the complexities of AI in data governance. Our solutions are designed to deliver accurate, reliable data that powers your AI strategies effectively. Reach out today for a complimentary consultation on fortifying your organization’s data quality for superior AI performance.