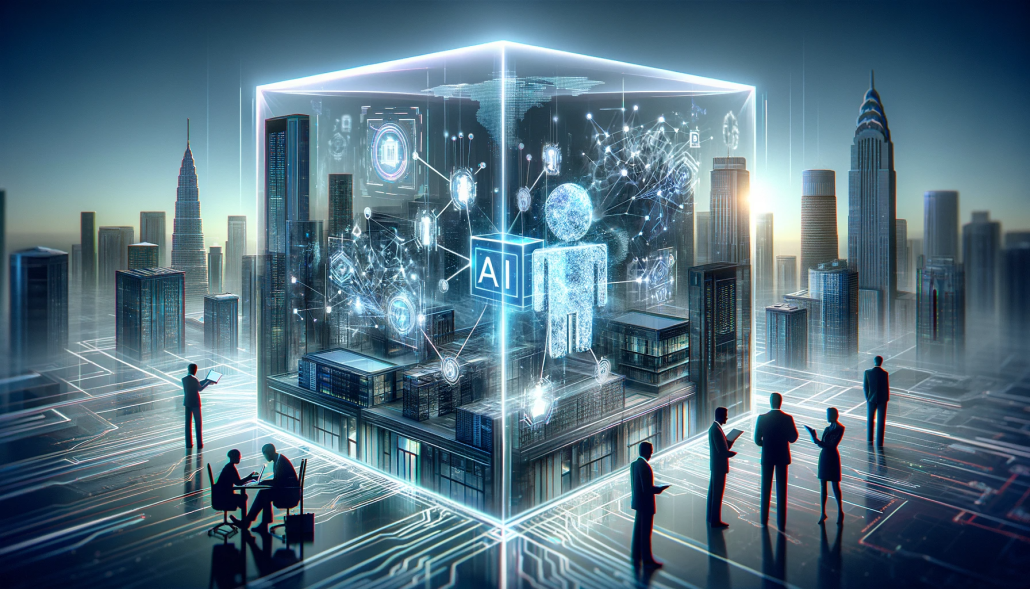
As Artificial Intelligence (AI) takes on a crucial role in Financial Services, concerns around the lack of transparency and accountability in AI systems have mounted. Deep learning models are often referred to as “black boxes” due to their complex inner workings, which can be challenging to understand and explain. In an industry where trust and clarity are paramount, the lack of transparency in these AI models can lead to significant problems. Moreover, when AI-driven processes don’t go as planned, pinpointing responsibility is a daunting task.
At Data Management Group (DMG), we understand these challenges and help financial institutions navigate this complex landscape. In this post of our series on how Financial Services companies can overcome the myriad challenges presented by deploying AI, we propose the following strategies and solutions to address these barriers and foster trust in AI systems:
- Strive to Implement Explainable AI – Ensure clarity around how your AI systems make high-stakes decisions
- Ensure Visibility into AI Processes – Provide clear documentation into how AI is being used
- Assign Accountability for AI Operations – Define who is in charge of managing the care and feeding of each AI system or technology deployed
Emphasizing the Importance of Explainable AI for High Stakes/Regulated Decisions
One key aspect of achieving transparency and accountability in AI is ensuring explainability for high stakes/regulated decisions. The people in charge of deploying AI models must be able to explain the AI models’ processes and decisions in a human-understandable format. This is important to fulfill regulatory requirements and also to build stakeholder trust and enable effective oversight. I wrote about the explainability imperative in one of the previous posts in this series, How Financial Services Companies Can Use AI While Maintaining Regulatory Compliance.
DMG’s Recommendations: Meet the explainability imperative in all your AI initiatives involving high stakes/regulated processes.
We recommend that Financial Services companies do the following:
- Prioritize Explainable AI (XAI) in their AI strategy. XAI models allow humans to understand, trust, and manage AI effectively. This includes models that provide clear reasoning for their decisions and models that avoid black box methods
- Invest in training initiatives for AI developers, decision-makers, and other relevant stakeholders. These initiatives should focus on how AI models function, how they make decisions, and how to interpret their outputs
- Use AI auditing tools that can help dissect the decision-making process of AI models. These can be particularly useful in verifying compliance and explaining the AI’s operations to regulators and stakeholders
- Leverage the expertise of third-party AI consultants to conduct regular AI audits, ensuring the models remain transparent and accountable over time
Enhance Visibility into AI Decision-Making Processes
Financial institutions must provide transparency into their AI-driven processes. This includes clear documentation of the AI model’s decision-making process, the data it uses, and the governance rules that apply. In essence, enhancing visibility into AI decision-making processes involves clarifying the ‘how,’ ‘what,’ and ‘who’ of AI systems: how they make decisions, what data they use, and who oversees and takes responsibility for them.
DMG’s Recommendations: Publish documentation around how your organization uses AI to drive decision-making.
We recommend that all Financial Services companies:
- Develop clear, comprehensive documentation about AI data usage, algorithms, and decision-making processes
- Implement advanced monitoring tools that provide real-time visibility into AI operations
- Regularly update stakeholders about AI deployments, their purposes, and their operations to foster trust and transparency
- Integrate AI systems with robust reporting tools to ensure all key activities and decisions are logged and reviewable
Assigning Accountability for AI Operations
To ensure accountability, it’s essential to define who is responsible for AI operations at various stages. This means defining who is responsible for the oversight, maintenance, and outputs of AI systems at various stages of their lifecycle – from development, validation, and deployment to ongoing management and auditing. Detailed protocols for handling AI anomalies, errors, or issues related to ethics and compliance are also crucial.
DMG’s Recommendations: Establish clear lines of accountability and responsibility at each stage of AI deployment.
We recommend that all Financial Services companies:
- Define clear, organization-wide roles and responsibilities for AI oversight, from development to deployment and management
- Establish protocols for handling anomalies and errors, including incident reporting and resolution procedures
- Create a cross-functional team that includes members from data science, legal, risk management, and business leaders to ensure diverse perspectives on AI accountability
- Craft detailed contingency plans for potential AI errors or failures, including communication strategies, technical backups, and resolution procedures
Overcoming the AI Transparency and Accountability Challenge
The “black box” nature of AI doesn’t have to be a barrier to its adoption in Financial Services. By prioritizing explainable AI for high stakes/regulated processes, enhancing visibility into AI processes, and assigning clear lines of accountability, financial institutions can build trust in their AI systems and use them to drive significant benefits. DMG is here to support your journey towards transparent and accountable AI. Contact us today for a complimentary consultation on how we can assist your organization in creating AI systems that are not just powerful but also transparent and accountable.