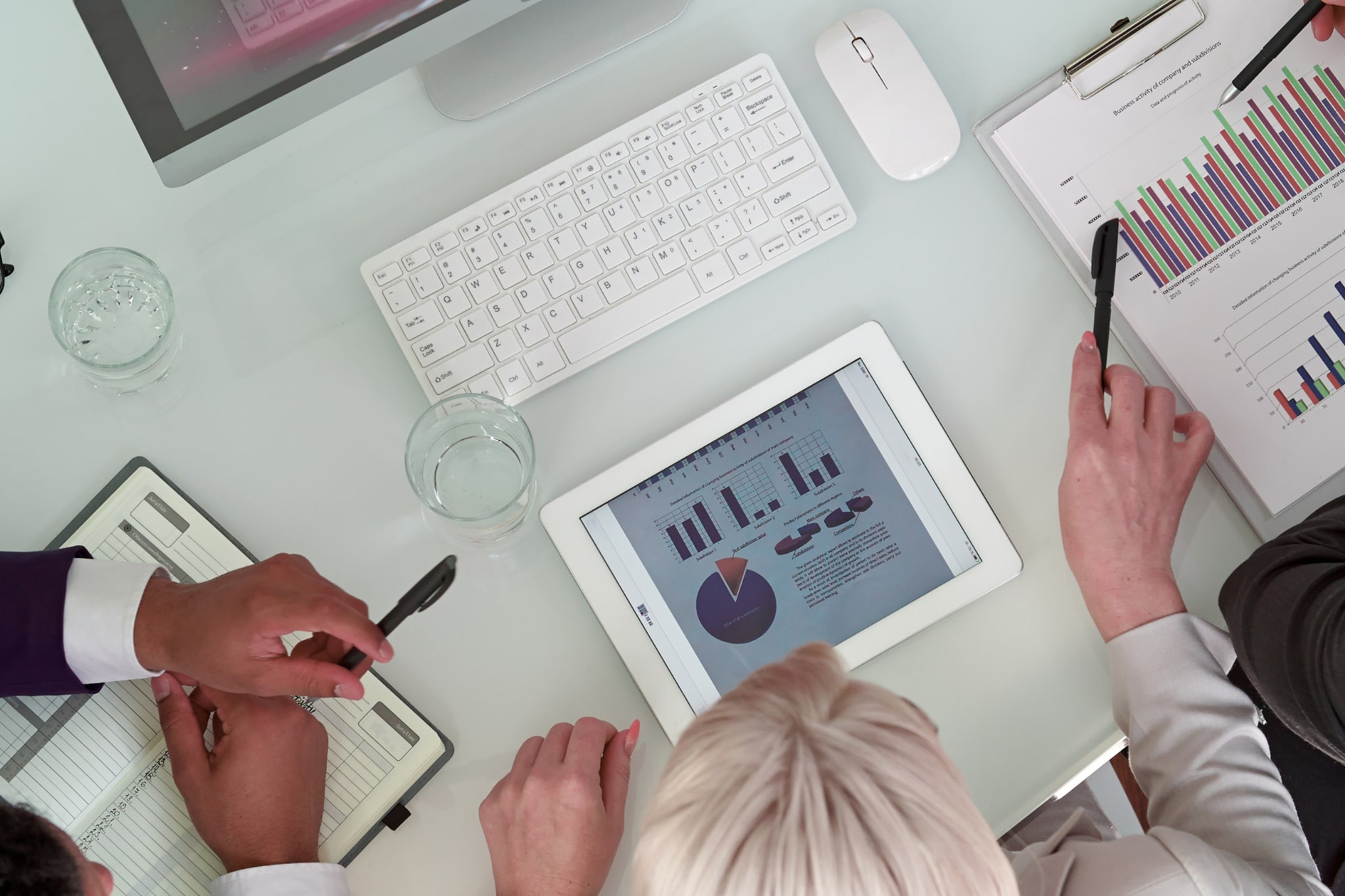
Since the days of Moneyball, many leaders have pushed their organizations to become increasingly data-driven. What few of these leaders fully grasp, however, is the additional workload this kind of fundamental shift places on existing staff. This is especially true when it comes to creating a high-functioning Data Governance model, which relies heavily on data quality and integrity to serve as the bedrock upon which business decisions can be made.
We frequently work with clients to establish strategies, approaches, and methods to ensure that they are operating with data of sufficient quality and integrity to establish a data-driven culture. Fortunately, there are a number of ways you can do so without adding substantial workload to your organization.
Many large organizations have a standard architecture approach which will need to be considered as part of Data Governance adoption. For example, some use The Open Group Architecture Framework (TOGAF) which is a high-level approach to design. It is typically modeled at four levels: Business, Application, Data, and Technology. If you are familiar with TOGAF or other frameworks, you will likely recognize these levels in the approach described here.
In this series of three blog posts, we will explore minimally invasive ways to ensure data quality and integrity in your Data Governance model without adding significant additional demands on your workforce.
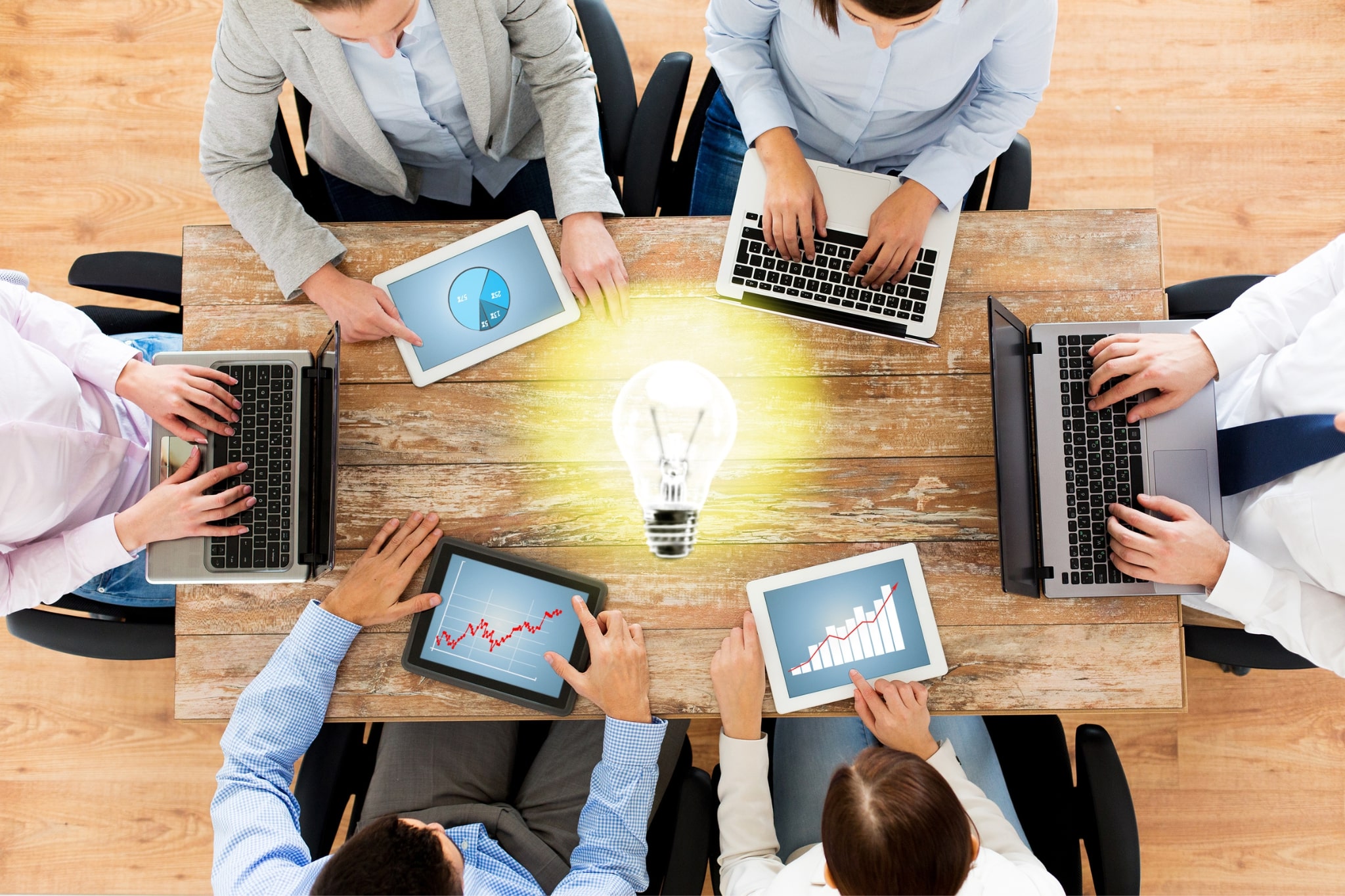
A Data Governance Strategy is a Must
One of the first steps in ensuring data quality and integrity is to have a clear Data Governance strategy in place. This strategy should outline the goals and objectives of your Data Governance program and provide a roadmap for achieving them.
Typical goals and objectives of a Data Governance program include:
- Data quality: Ensuring that data is accurate, complete, and consistent across different systems and departments.
- Data security: Protecting data from unauthorized access, use, and disclosure.
- Data privacy: Ensuring that data is collected, processed, and used in compliance with privacy laws and regulations.
- Data management: Developing policies and procedures for the creation, use, storage, and disposal of data.
- Data integration: Facilitating the integration of data from different sources and systems to improve decision-making and operational efficiency.
- Data standardization: Establishing common standards and definitions for data elements to improve consistency and reduce errors.
- Data accessibility: Ensuring that data is available to authorized users when and where it is needed.
- Data Governance management and oversight: Monitoring compliance with Data Governance policies and procedures, and identifying areas for improvement.
- Data Governance education: Providing education and training to employees on the importance of Data Governance and their roles and responsibilities in maintaining data quality, security, and privacy.
- Data Governance culture: Creating a culture of Data Governance throughout the organization to ensure that data is valued and managed as a strategic asset.
A Data Governance strategy will help you identify the data sources that are most critical to your business, determine the level of data quality required for each source, and establish processes for ensuring that data quality is maintained.
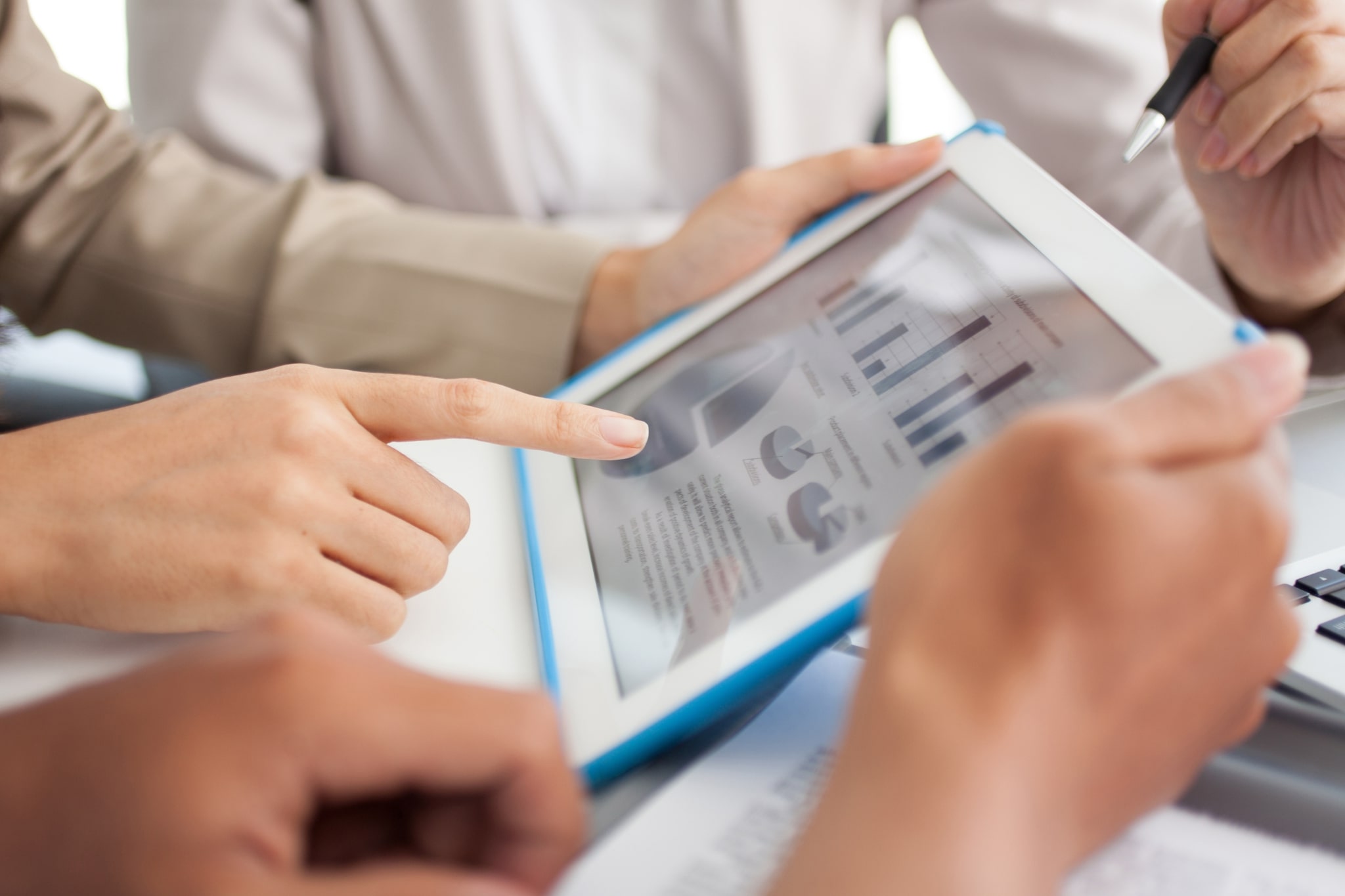
Measuring Business Value – Quantifying Success and Improvement
One of the significant challenges in standing up a data governance program is the daunting amount of data that organizations today have. It seems overwhelming…so where do you start?
In our experience there is some set of data that has more business value than other data. Sometimes we call this critical data. Critical data elements might include: key transactional data, financial data, or regulated data (e.g. PII, HIPAA, etc). Part of the Data Governance strategy and charter will define the critical data and areas that the program needs to impact. The success metrics need to be aligned with the strategy, and this may include metrics for the handling, quality, and security of these data elements.
Additionally, the Data Governance program itself will have metrics associated with it. These may include key milestones such as when the Data Governance Charter and Policy were approved, how many systems are in the adoption phase of Data Governance, and when the initial set of critical data were identified.
The bottom line is that Metrics Drive Behavior, and since Data Governance is largely about modifying the organization’s behavior regarding data, we must have a set of initial (and improving) metrics to understand the impact of the Data Governance program and the value to the organization.
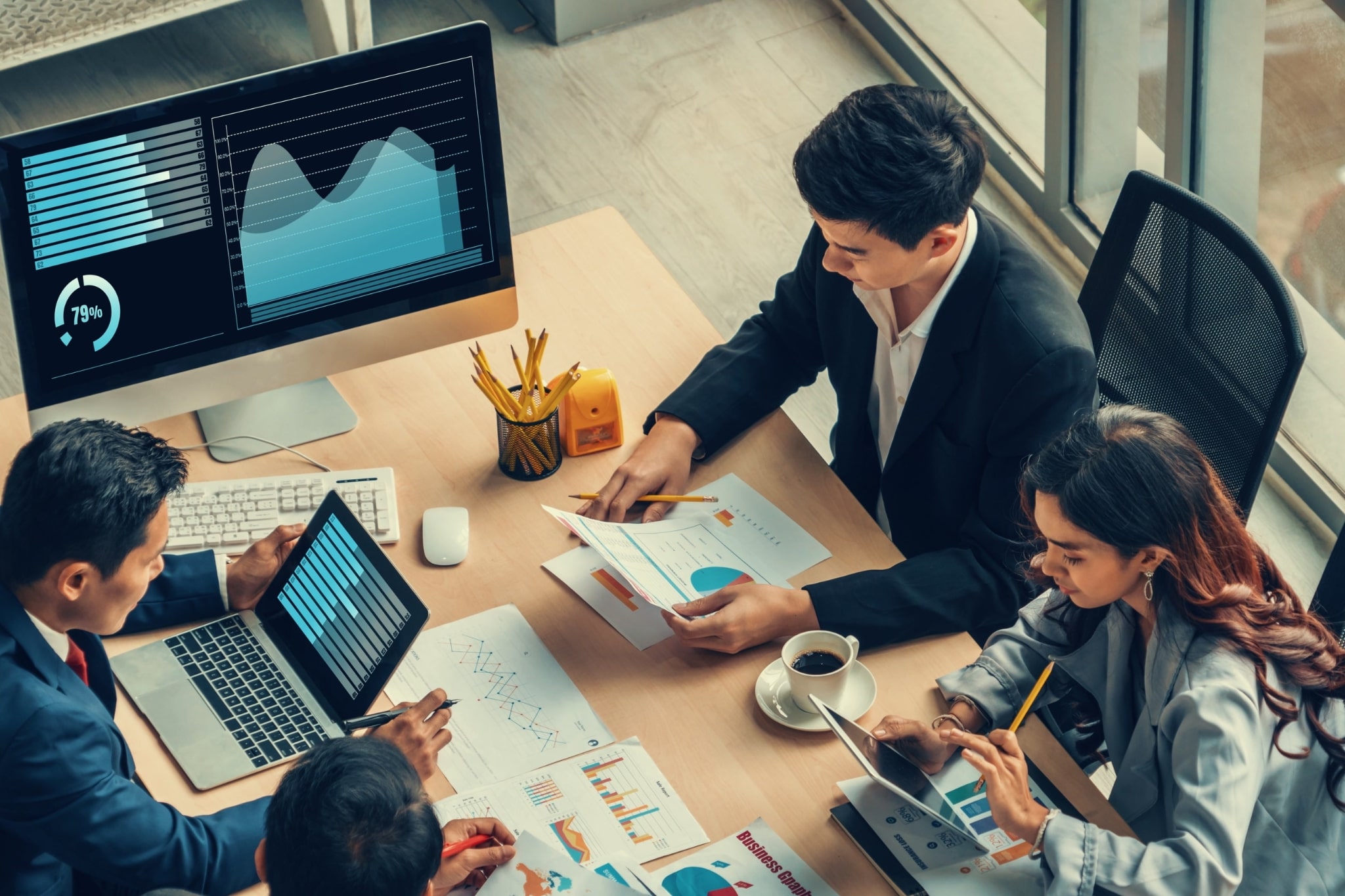
Data Governance Tools are Key To Enabling and Accelerating Efforts
Once a Data Governance strategy is in place, you’ll want to evaluate which tools and technologies are needed to delivery on your strategy and roadmap. Technology is undoubtedly a cornerstone of getting your Data Governance efforts firing on all cylinders without placing huge additional demands on your team members.
There are a wide variety of tools that DMG has used to help enterprises elevate their Data Governance efforts. These include:
- Data Quality Management tools like Informatica Data Quality and Talend Data Quality; and AI tools such as DataRobot, Trifacta, and DataWrangler by Google Cloud
- BI tools like Qlik, Tableau, and PowerBI
- ETL tools like Talend, Microsoft SSIS, and Oracle Data Integrator
- Data Governance tools like Collibra, Alation, and BigID
- Cloud-based data quality services like Microsoft Azure Data Factory and AWS Data Quality Services.
There are many other tools and methods available. These are representative examples. DMG is partners with or certified in a number of Data Governance tools and technologies. We regularly assist customers with optimization of their existing tools and selection and implementation of new tools based on their Data Governance strategy.
Let’s Consider Implementation Best Practices
Once a Data Governance strategy, success metrics, and the requisite Data Governance tools are in place, it’s time to move on to the most rewarding parts of your Data Governance efforts: implementation, execution, and iteration. In our next two posts, we’ll look at best practices we guide customers through to ensure their Data Governance efforts are successful with minimal impact to their teams’ workload.
You can learn more about our lean approach to Data Governance and schedule a complimentary consultation today if your organization would like outside expertise formalizing or improving your existing data governance approach.