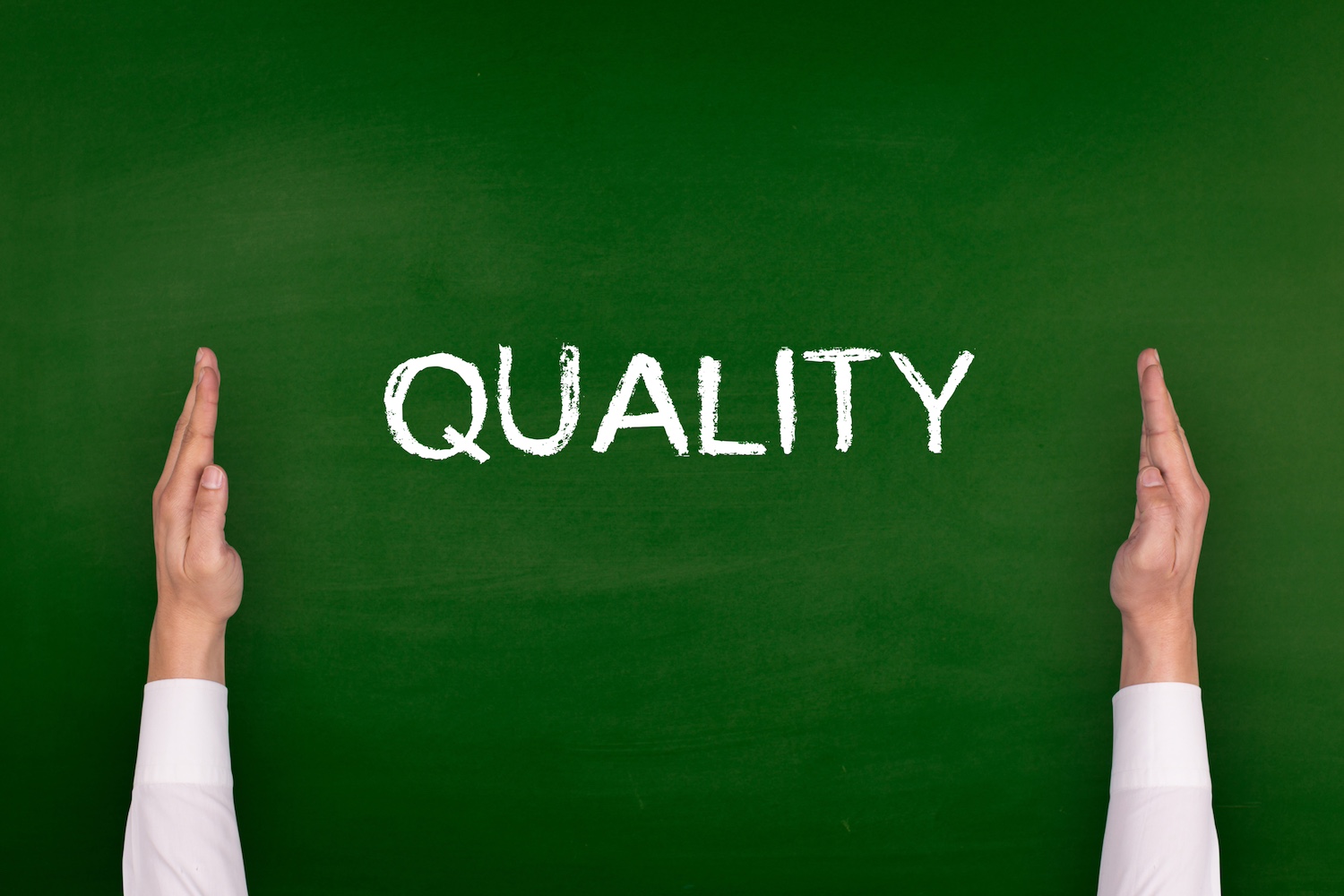
Data Governance Best Practices
Formalizing and improving your Data Governance framework is one of the soundest ways to ensure that your company does more than just pay lip service to being data-driven. In this post, I’ll cover two Data Governance best practices that can set you well on your way to what I called in a previous post A Minimally Invasive Approach to Formalizing or Improving Data Governance.
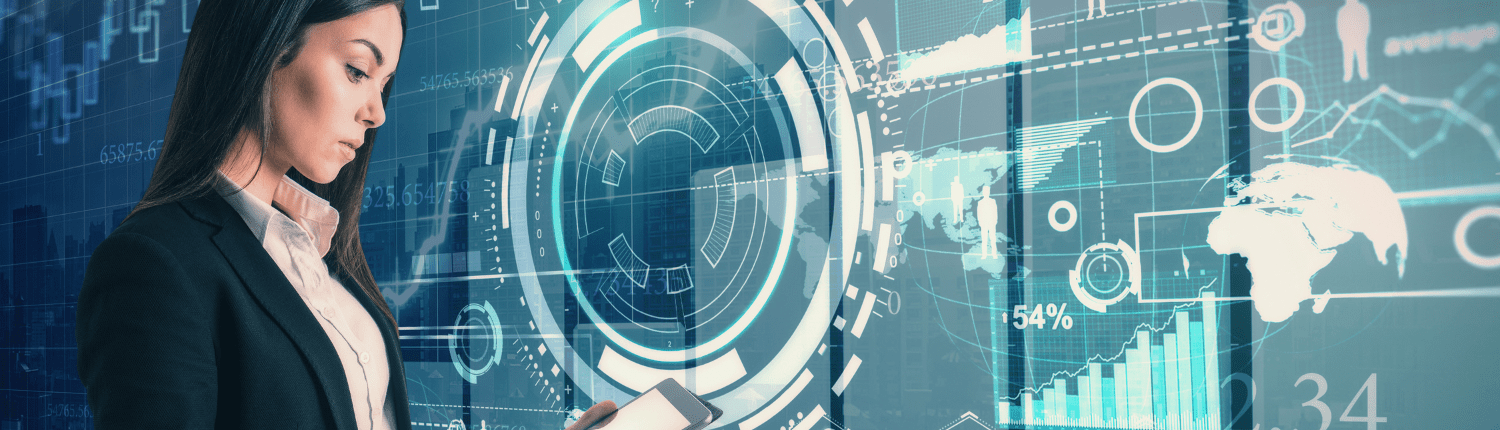
Why You Need to Establish Data Quality Metrics
As we have mentioned previously, Data Governance is largely about modifying peoples’ behavior with relation to data. Metrics drive behavior, and are critical to the establishment and success of Data Governance programs.
Most Data Governance programs are concerned about Data Quality, so let’s delve a little deeper into Data Quality metrics. Data quality metrics are important to measure the effectiveness of your Data Governance program and ensure that data quality and integrity are maintained. By establishing data quality metrics, you can make adjustments as needed to ensure that data quality is maintained.
These are some common data quality metrics that DMG frequently helps companies measure:
- Completeness measures the percentage of required data fields that are populated in a dataset. A company might measure the completeness of customer records to ensure that all required fields are present.
- Accuracy measures the degree to which data reflects the actual state of the real-world object or event it represents. You likely want to measure the accuracy of sales data, for example, to ensure that it reflects the actual sales transactions that occurred.
- Consistency measures the degree to which data is consistent across different sources or systems. A company could measure the consistency of product data across different sales channels to ensure that customers receive consistent and accurate product information.
- Timeliness measures the degree to which data is up-to-date and reflects the current state of the business. The timeliness of financial data to ensure that reports and analyses are based on the most recent data available would be an example of such a measure.
- Validity measures the degree to which data conforms to predefined business rules and standards. You could measure the validity of customer data to ensure that it conforms to established data quality standards.
- Relevance measures the degree to which data is useful and applicable for the intended purpose, like the relevance of marketing data to ensure that it is applicable to the target audience and can be used to make effective marketing decisions.
- Duplication measures the number of duplicate records in a dataset. Duplicate data can cause inaccuracies and inconsistencies in data analyses and reporting.
- Integrity measures the degree to which data is complete, accurate, and consistent over time. A company might measure the integrity of employee data to ensure that it remains accurate and up-to-date throughout the employee lifecycle.
Automated data quality checks can be implemented to run in the background so they identify any issues with data quality in real-time. DMG recommends at minimum setting up rules that check for missing data, inconsistent data, and duplicate records. If and when these issues can be caught early, they can be flagged for troubleshooting before they’re allowed to permeate and erode data quality.
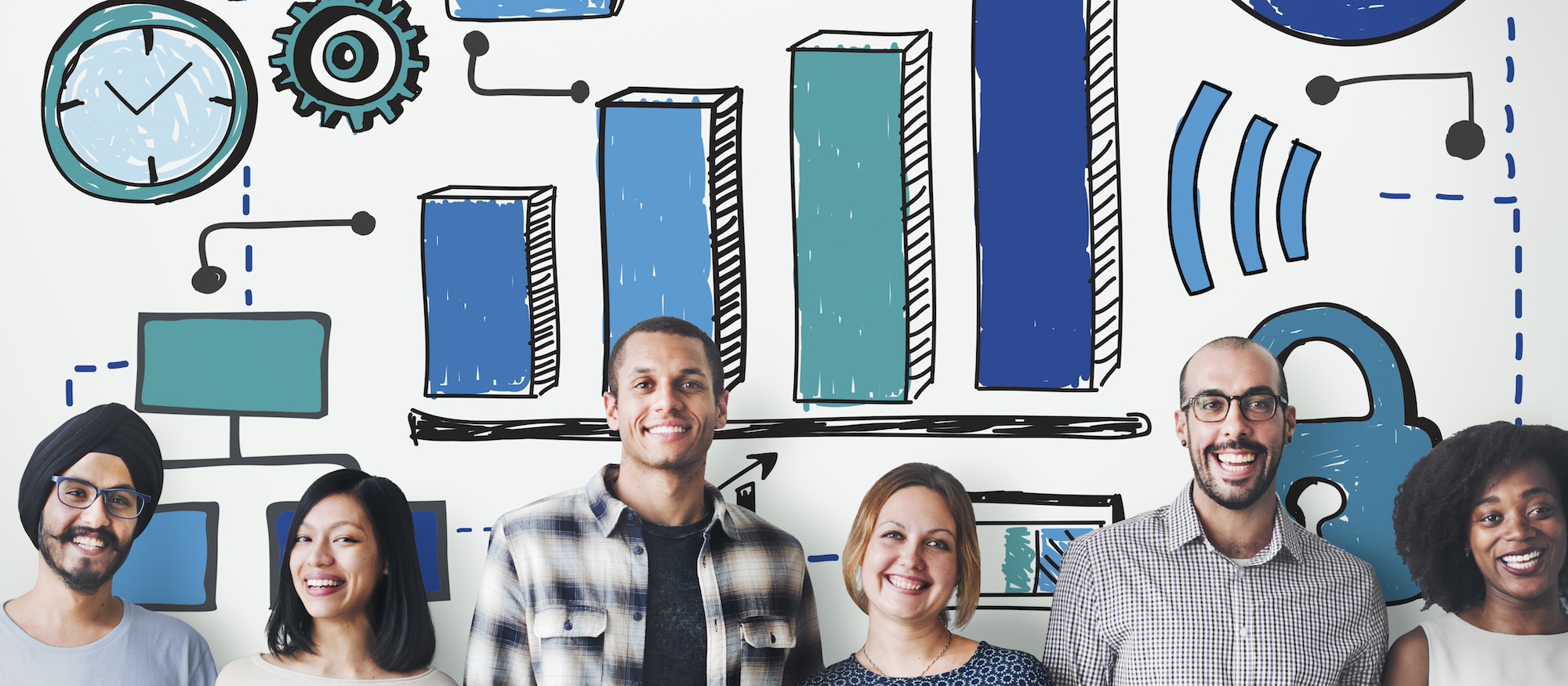
Develop a Data Quality Culture
Developing a data quality culture is perhaps the most important aspect of ensuring data quality and integrity. A data quality culture is one in which all members of the workforce understand the importance of data quality and are committed to maintaining it.
Just a few of the ways you can do this include establishing data quality goals, objectives, and metrics; providing regular feedback to your team on data quality metrics and measurable impact your Data Governance efforts are having on the business; and recognizing and rewarding individuals or teams that contribute to maintaining data quality. By establishing a data quality culture, you can ensure that data quality and integrity become tightly intertwined with your overall approach to Data Governance.
Capturing the Benefits of Data Governance Doesn’t Have to Be Painful
Implementing a robust Data Governance program at the enterprise level can be challenging, but there are a number of strategies you can take to ensure you minimize the additional strain placed on your team. This is an area where we have successfully led numerous transformations for enterprise clients to help them go from questioning their data to having their data help answer some of their most vexing questions.
If you haven’t read the first 2 posts in this series yet, you can read ‘A Minimally Invasive Approach to Formalizing or Improving Data Governance’ here and ‘Automated Data Quality Checks and Standardized Data Formats’ here. You can learn more about our lean approach to Data Governance and schedule a complimentary consultation today if your organization would like outside expertise formalizing or improving your existing Data Governance approach.